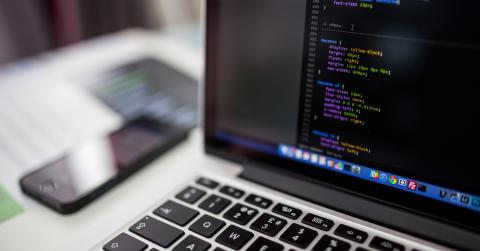
Sort our Insights
Content-Type
Technological Partner
Topic
Industry
Highlights of the Oracle Enterprise Data Management 24.03 release
For a while now, Oracle has developed a cadence with the monthly releases of Oracle Enterprise Data Management (EDM). Ab...
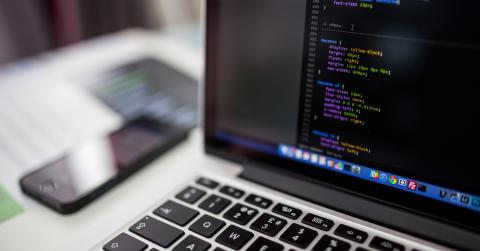
Apr 30 - May 2 - Microsoft 365 Community Conference
Alithya is a proud exhibitor at the Microsoft 365 Community Conference on April 30 – May 2 in Orlando, Florida! ...
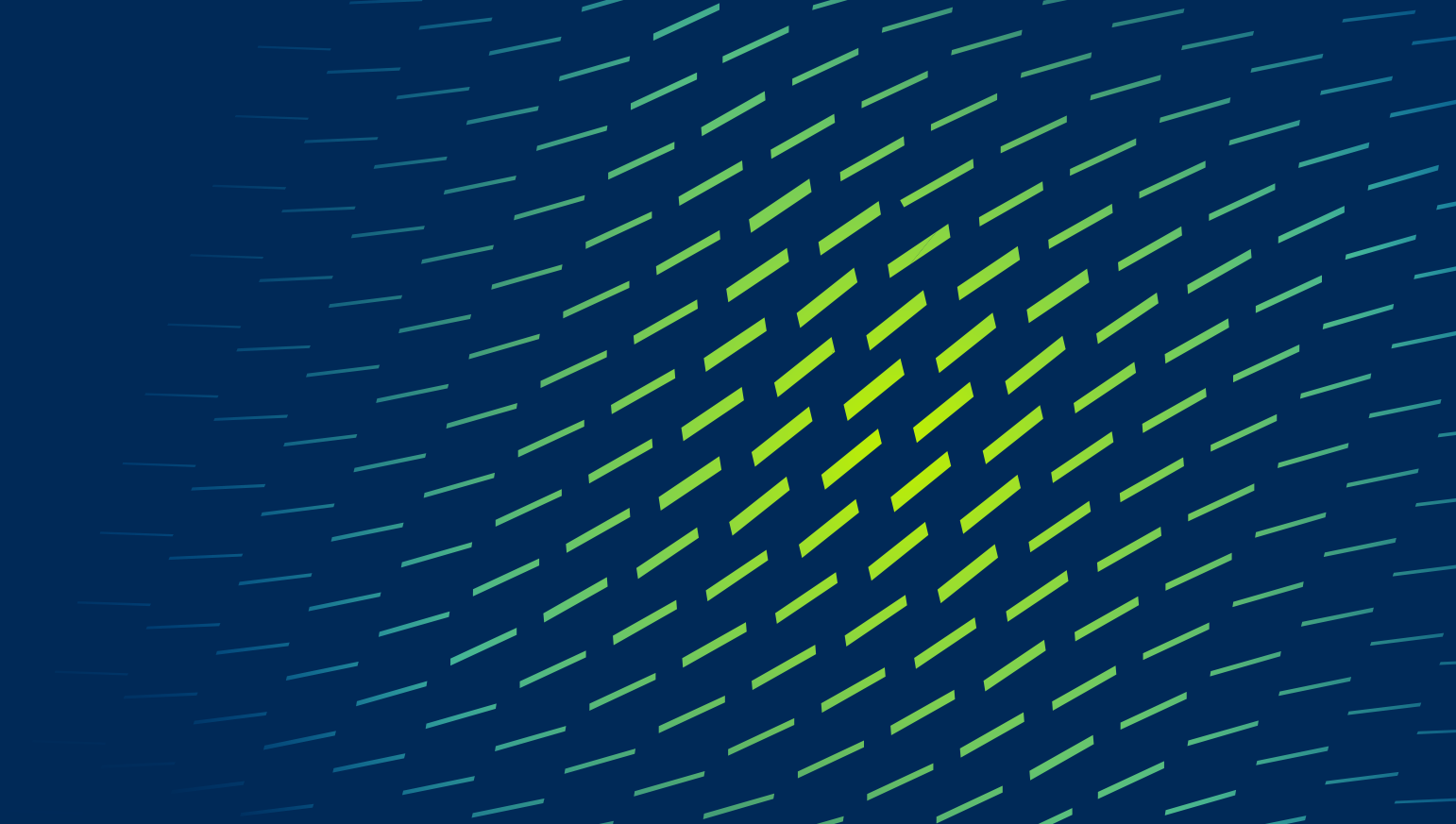
May 8 - 2024 TMX Equities Trading Conference
Alithya invites you to the 20th Annual TMX Equities Trading Conference on Wednesday, May 8th in Toronto. Engage with tra...
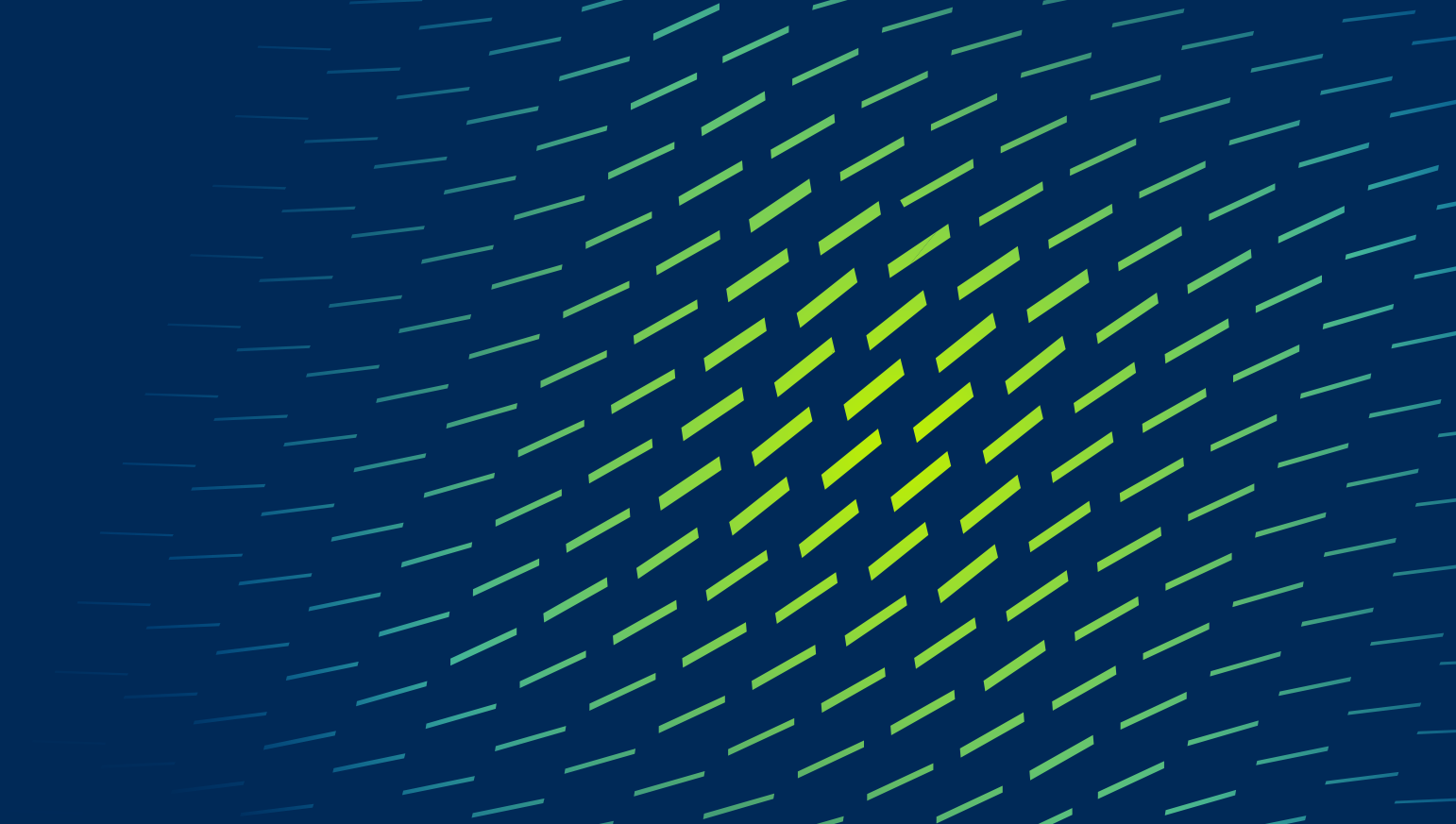
Simplify and accelerate app migrations to the cloud
As part of your cloud migration strategy, you’ve spent months or years meticulously setting up your ...
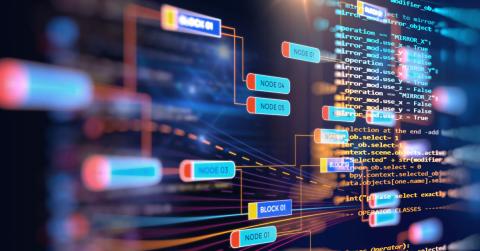
Tackling FinOps and cloud cost optimization
Have you ever received a huge power bill at the end of a really hot month, or a huge gas or electricity bill at the end ...
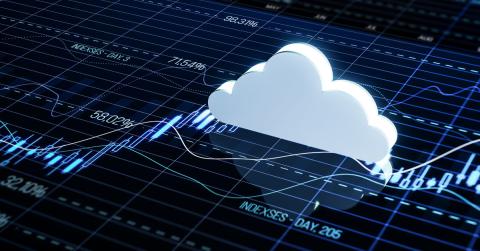
Embracing a secure multi-cloud strategy
Consider these two shocking statistics: More than 85% of businesses have embraced multi-cloud...
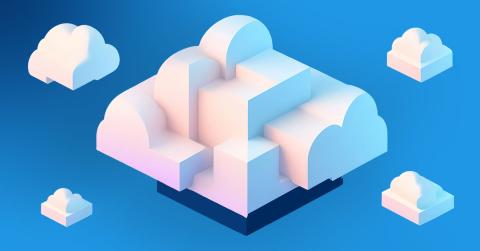
Jun 17-20 - Acsend 2024
Ascend is the Oracle user community event that unites functional users, IT professionals, and experts for frank discussi...
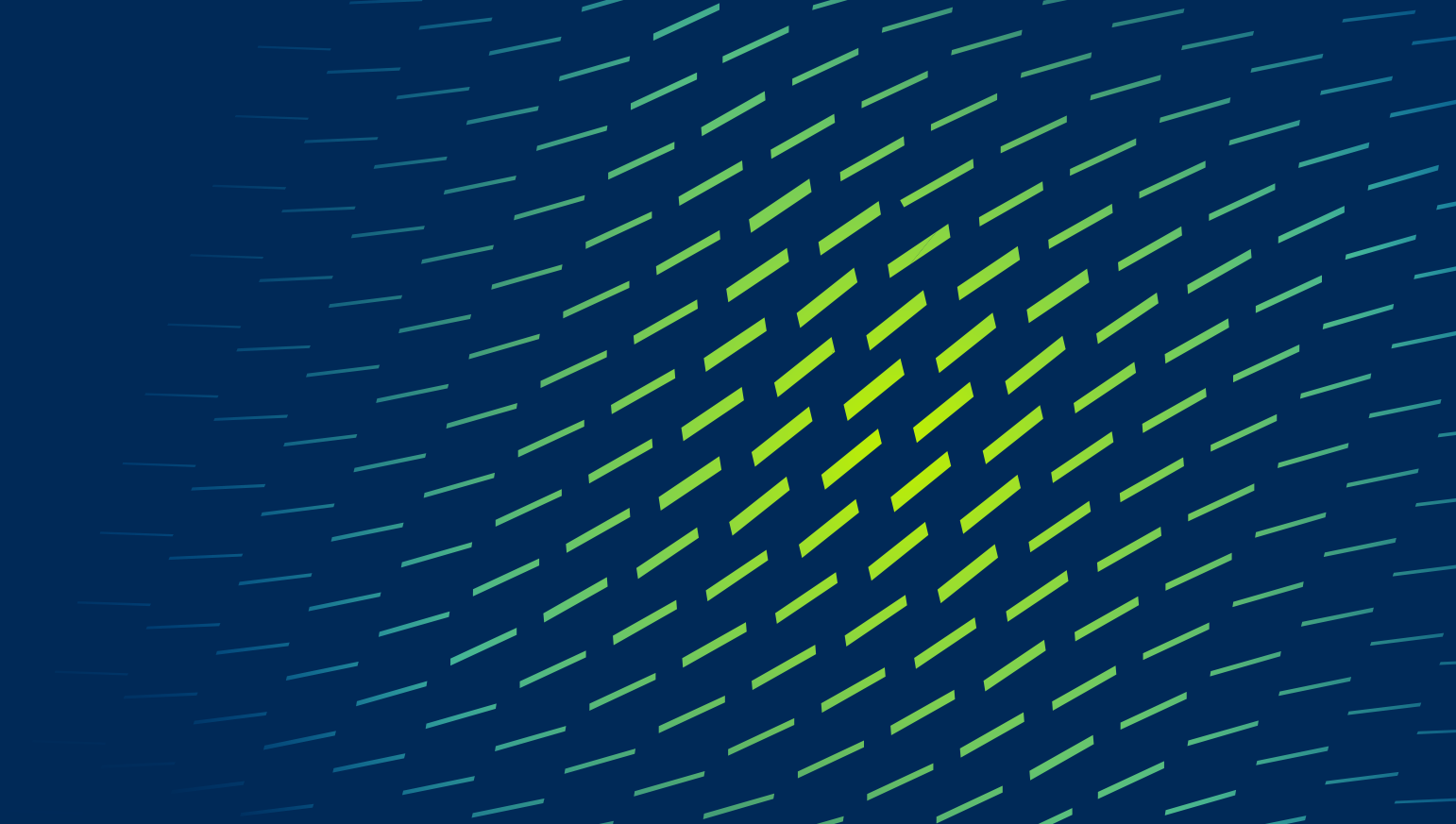
How Azure is empowering financial services transformation
For competitive financial services institutions, digital transformation is no longer an option, it’s a necessity....
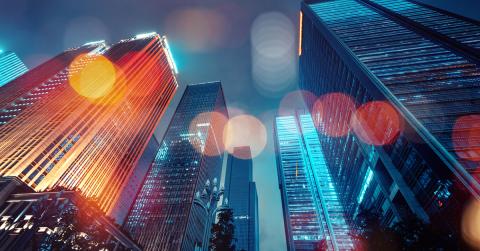
Microsoft AI Copilot – the third technological revolution
Over three decades ago, a historic moment forever altered the future of technology. The momentous occasion? The birth of...
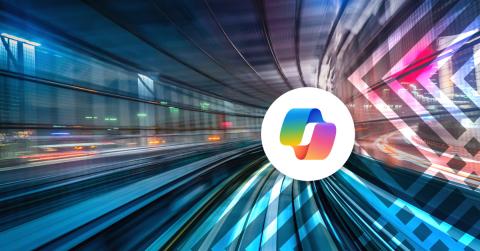
Decoding Dynamics 365: your essential guide to Microsoft's CRM
Customer relationship management (CRM) is a strategy for managing an organization's interactions with current an...
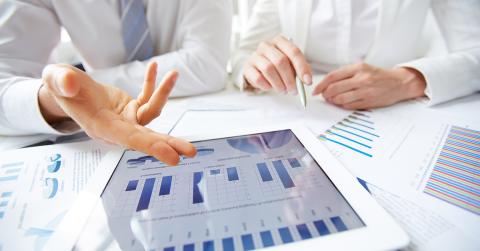
Unleashing the power of Microsoft Dynamics 365 CRM with AI
In the ever-evolving landscape of customer relationship management (CRM), the pursuit of the ideal system is a j...

Alithya welcomes Ines Gbegan to its Board of Directors
MONTREAL, March 21, 2024 /CNW/ - The Board of Directors of Alithya Group Inc. (TSX: ALYA) ("Alithya") today announced th...
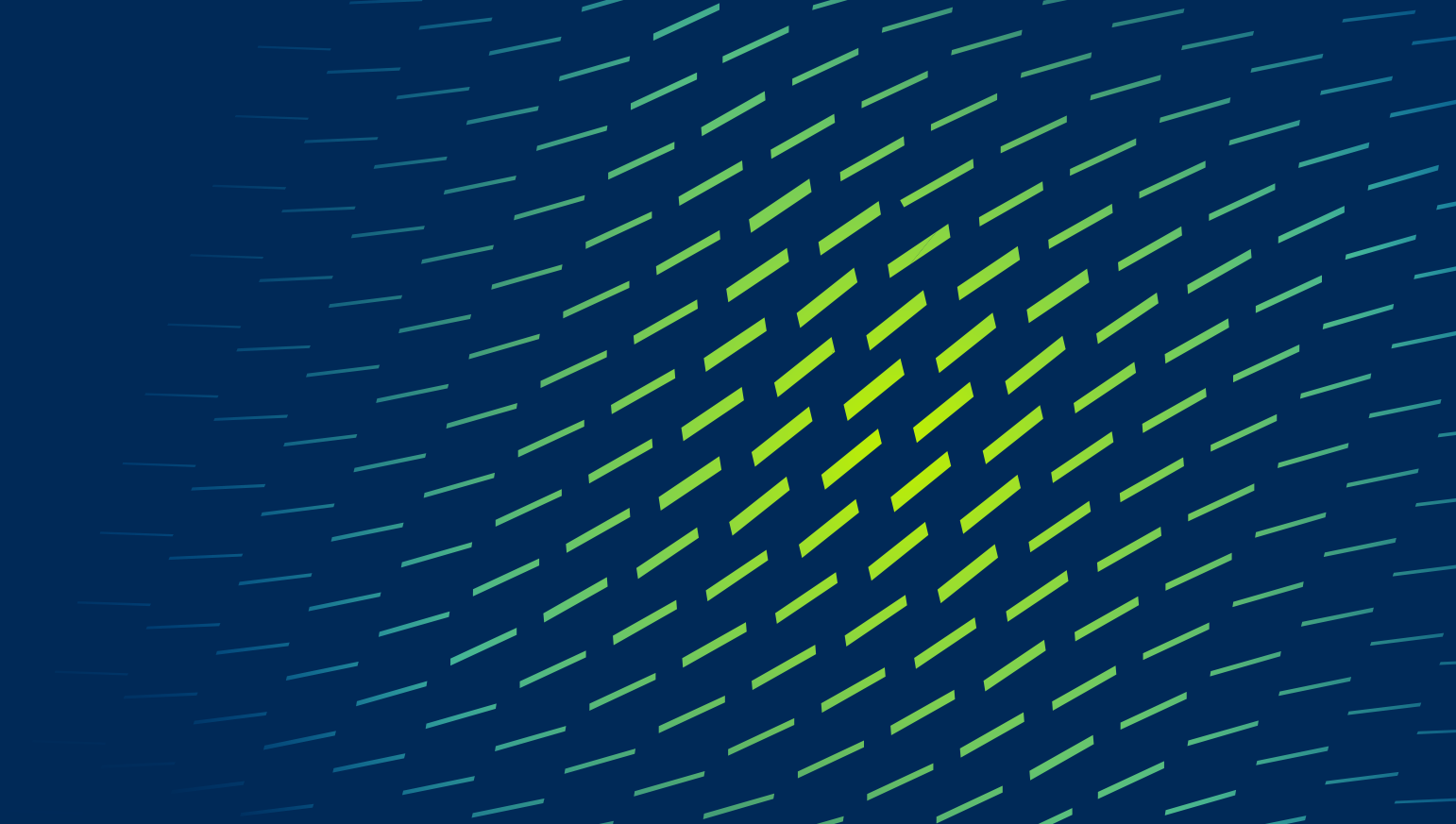